Schneider Electric – Schneider Electric Issues Industry-First Blueprint for Optimizing Data Centers to Harness the Power of Artificial Intelligence
Data Centers Must Adapt Physical Infrastructure Design to Meet Evolving Needs in the Era of Artificial Intelligence (AI) Disruption
Schneider Electric, the leader in the digital transformation of energy management and automation, launched today an industry-first guide to addressing new physical infrastructure design challenges for data centers to support the shift in artificial intelligence (AI)-driven workloads, setting the gold standard for AI-optimized data center design.
Titled “The AI Disruption: Challenges and Guidance for Data Center Design,” this groundbreaking document provides invaluable insights and acts as a comprehensive blueprint for organizations seeking to leverage AI to its fullest potential within their data centers, including a forward-looking view of emerging technologies to support high density AI clusters in the future.
Artificial Intelligence disruption has brought about significant changes and challenges in data center design and operation. As AI applications have become more prevalent and impactful on industry sectors ranging from healthcare and finance to manufacturing, transportation and entertainment, so too has the demand for processing power. Data centers must adapt to meet the evolving power needs of AI-driven applications effectively.
Pioneering the Future of Data Center Design
AI workloads are projected to grow at a compound annual growth rate (CAGR) of 26-36% by 2028, leading to increased power demand within existing and new data centers. Servicing this projected energy demand involves several key considerations outlined in the White Paper, which addresses the four physical infrastructure categories – power, cooling, racks and software tools. White Paper 110 is available for download here.
In an era where AI is reshaping industries and redefining competitiveness, Schneider Electric’s latest white paper paves the way for businesses to design data centers that are not just capable of supporting AI, but fully optimized for it. The white paper introduces innovative concepts and best practices, positioning Schneider Electric as a frontrunner in the evolution of data center infrastructure.
“As AI continues to advance, it places unique demands on data center design and management. To address these challenges, it’s important to consider several key attributes and trends of AI workloads that impact both new and existing data centers,” said Pankaj Sharma, Executive Vice President, Secure Power Division at Schneider Electric. “AI applications, especially training clusters, are highly compute-intensive and require large amounts of processing power provided by GPUs or specialized AI accelerators. This puts a significant strain on the power and cooling infrastructure of data centers. And as energy costs rise and environmental concerns grow, data centers must focus on energy-efficient hardware, such as high-efficiency power and cooling systems, and renewable power sources to help reduce operational costs and carbon footprint.”
This new blueprint for organizations seeking to leverage AI to its fullest potential within their data centers, has received welcome support from customers.
“The AI market is fast-growing and we believe it will become a fundamental technology for enterprises to unlock outcomes faster and significantly improve productivity,” said Evan Sparks, chief product officer for Artificial Intelligence, at Hewlett Packard Enterprise. “As AI becomes a dominant workload in the data center, organizations need to start thinking intentionally about designing a full stack to solve their AI problems. We are already seeing massive demand for AI compute accelerators, but balancing this with the right level of fabric and storage and enabling this scale requires well-designed software platforms. To address this, enterprises should look to solutions such as specialized machine learning development and data management software that provide visibility into data usage and ensure data is safe and reliable before deploying. Together with implementing end-to-end data center solutions that are designed to deliver sustainable computing, we can enable our customers to successfully design and deploy AI, and do so responsibly.”
Unlocking the Full Potential of AI
Schneider Electric’s AI-Ready Data Center Guide explores the critical intersections of AI and data center infrastructure, addressing key considerations such as:
- Guidance on the four key AI attributes and trends that underpin physical infrastructure challenges in power, cooling, racks and software management
- Recommendations for assessing and supporting the extreme rack power densities of AI training servers.
- Guidance for achieving a successful transition from air cooling to liquid cooling to support the growing thermal design power (TDP) of AI workloads.
- Proposed rack specifications to better accommodate AI servers that require high power, cooling manifolds and piping, and large number of network cables.
- Guidance on using data center infrastructure management (DCIM), electrical power management system (EPMS) and building management system (BMS) software for creating digital twins of the data center, operations and asset management.
- Future outlook of emerging technologies and design approaches to help address AI evolution.
For more information about Schneider Electric’s AI data center solutions and expertise, please visit Schneider Electric.
SourceSchneider Electric
EMR Analysis
More information on Schneider Electric: See the full profile on EMR Executive Services
More information on Peter Herweck (Chief Executive Officer, Schneider Electric): See the full profile on EMR Executive Services
More information on Pankaj Sharma (Executive Vice President, Secure Power, Schneider Electric): See the full profile on EMR Executive Services
More information on Industry Guide “The AI Disruption: Challenges and Guidance for Data Center Design” by Schneider Electric: https://www.se.com/ww/en/download/document/SPD_WP110_EN/?=1 + From large training clusters to small edge inference servers, AI is becoming a larger percentage of data center workloads. This represents a shift to higher rack power densities. AI start-ups, enterprises, colocation providers, and internet giants must now consider the impact of these densities on the design and management of the data center physical infrastructure. This paper explains relevant attributes and trends of AI workloads, and describes the resulting data center challenges. Guidance to address these challenges is provided for each physical infrastructure category including power, cooling, racks, and software management.
More information on Hewlett Packard Enterprise (HPE): https://www.hpe.com/us/en/home.html +HPE is the global edge-to-cloud company built to transform your business. How? By helping you connect, protect, analyze, and act on all your data and applications wherever they live, from edge to cloud, so you can turn insights into outcomes at the speed required to thrive in today’s complex world.
More information on Antonio Neri (President & Chief Executive Officer, Hewlett Packard Enterprise): https://www.hpe.com/us/en/leadership-bios/antonio-neri.html + https://www.linkedin.com/in/antonio-neri-hpe/
EMR Additional Notes:
- Blueprint:
- A blueprint is a guide for making something — it’s a design or pattern that can be followed. Want to build the best tree house ever? Draw up a blueprint and follow the design carefully. The literal meaning of a blueprint is a paper — which is blue — with plans for a building printed on it.
- After the paper was washed and dried to keep those lines from exposing, the result was a negative image of white (or whatever color the blueprint paper originally was) against a dark blue background. The resulting image was therefore appropriately named “blueprint.”.
- By definition, a blueprint is a drawing up of a plan or model. The blueprint perspective allows you to see all the pieces needed to assemble your business before you begin.
- AI – Artificial Intelligence:
- https://searchenterpriseai.techtarget.com/definition/AI-Artificial-Intelligence +
- Artificial intelligence is the simulation of human intelligence processes by machines, especially computer systems. Specific applications of AI include expert systems, natural language processing, speech recognition and machine vision.
- As the hype around AI has accelerated, vendors have been scrambling to promote how their products and services use AI. Often what they refer to as AI is simply one component of AI, such as machine learning. AI requires a foundation of specialized hardware and software for writing and training machine learning algorithms. No one programming language is synonymous with AI, but a few, including Python, R and Java, are popular.
- In general, AI systems work by ingesting large amounts of labeled training data, analyzing the data for correlations and patterns, and using these patterns to make predictions about future states. In this way, a chatbot that is fed examples of text chats can learn to produce lifelike exchanges with people, or an image recognition tool can learn to identify and describe objects in images by reviewing millions of examples.
- AI programming focuses on three cognitive skills: learning, reasoning and self-correction.
- What are the 4 types of artificial intelligence?
- Type 1: Reactive machines. These AI systems have no memory and are task specific. An example is Deep Blue, the IBM chess program that beat Garry Kasparov in the 1990s. Deep Blue can identify pieces on the chessboard and make predictions, but because it has no memory, it cannot use past experiences to inform future ones.
- Type 2: Limited memory. These AI systems have memory, so they can use past experiences to inform future decisions. Some of the decision-making functions in self-driving cars are designed this way.
- Type 3: Theory of mind. Theory of mind is a psychology term. When applied to AI, it means that the system would have the social intelligence to understand emotions. This type of AI will be able to infer human intentions and predict behavior, a necessary skill for AI systems to become integral members of human teams.
- Type 4: Self-awareness. In this category, AI systems have a sense of self, which gives them consciousness. Machines with self-awareness understand their own current state. This type of AI does not yet exist.
- Machine Learning:
- Developed to mimic human intelligence. It lets the machines learn independently by ingesting vast amounts of data and detecting patterns. Many ML algorithms use statistics formulas and big data to function.
- Type of artificial intelligence (AI) that allows software applications to become more accurate at predicting outcomes without being explicitly programmed to do so. Machine learning algorithms use historical data as input to predict new output values.
- Recommendation engines are a common use case for machine learning. Other popular uses include fraud detection, spam filtering, malware threat detection, business process automation (BPA) and Predictive maintenance.
- Classical machine learning is often categorized by how an algorithm learns to become more accurate in its predictions. There are four basic approaches:supervised learning, unsupervised learning, semi-supervised learning and reinforcement learning. The type of algorithm data scientists choose to use depends on what type of data they want to predict.
- Deep Learning:
- Subset of machine learning. Deep learning enabled much smarter results than were originally possible with machine learning. Consider the face recognition example.
- Deep learning makes use of layers of information processing, each gradually learning more and more complex representations of data. The early layers may learn about colors, the next ones learn about shapes, the following about combinations of those shapes, and finally actual objects. Deep learning demonstrated a breakthrough in object recognition.
- Deep learning is currently the most sophisticated AI architecture we have developed.
- Computer Vision:
- Computer vision is a field of artificial intelligence (AI) that enables computers and systems to derive meaningful information from digital images, videos and other visual inputs — and take actions or make recommendations based on that information.
- The most well-known case of this today is Google’s Translate, which can take an image of anything — from menus to signboards — and convert it into text that the program then translates into the user’s native language.
- https://searchenterpriseai.techtarget.com/definition/AI-Artificial-Intelligence +
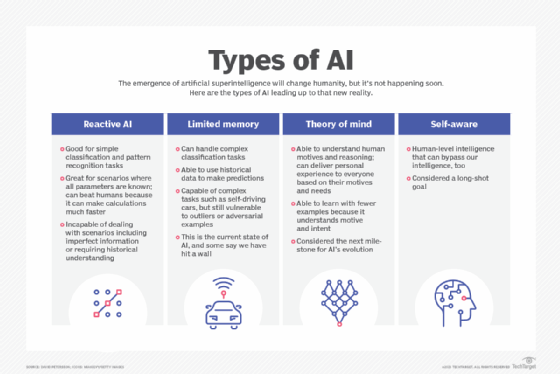
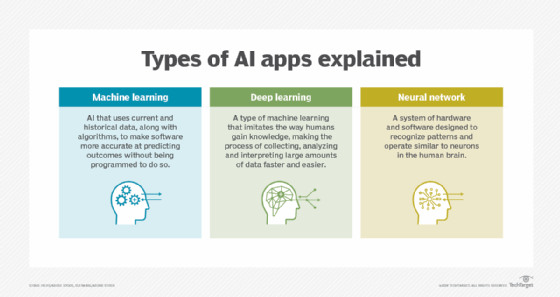
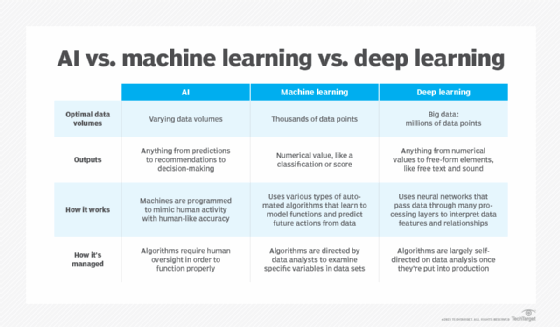
- Cloud Computing:
- Cloud computing is a general term for anything that involves delivering hosted services over the internet. … Cloud computing is a technology that uses the internet for storing and managing data on remote servers and then access data via the internet.
- Cloud computing is the on-demand availability of computer system resources, especially data storage and computing power, without direct active management by the user. Large clouds often have functions distributed over multiple locations, each location being a data center.
- Edge Computing:
- Edge computing is a form of computing that is done on site or near a particular data source, minimizing the need for data to be processed in a remote data center.
- Edge computing can enable more effective city traffic management. Examples of this include optimising bus frequency given fluctuations in demand, managing the opening and closing of extra lanes, and, in future, managing autonomous car flows.
- An edge device is any piece of hardware that controls data flow at the boundary between two networks. Edge devices fulfill a variety of roles, depending on what type of device they are, but they essentially serve as network entry — or exit — points.
- There are five main types of edge computing devices: IoT sensors, smart cameras, uCPE equipment, servers and processors. IoT sensors, smart cameras and uCPE equipment will reside on the customer premises, whereas servers and processors will reside in an edge computing data centre.
- In service-based industries such as the finance and e-commerce sector, edge computing devices also have roles to play. In this case, a smart phone, laptop, or tablet becomes the edge computing device.
- Data Centers:
- A data center is a facility that centralizes an organization’s shared IT operations and equipment for the purposes of storing, processing, and disseminating data and applications. Because they house an organization’s most critical and proprietary assets, data centers are vital to the continuity of daily operations.
- Hyperscale Data Centers:
- The clue is in the name: hyperscale data centers are massive facilities built by companies with vast data processing and storage needs. These firms may derive their income directly from the applications or websites the equipment supports, or sell technology management services to third parties.
- Graphic Processing Unit (GPU):
- Computer chip that renders graphics and images by performing rapid mathematical calculations. GPUs are used for both professional and personal computing. Traditionally, GPUs are responsible for the rendering of 2D and 3D images, animations and video — even though, now, they have a wider use range.
- A GPU may be found integrated with a CPU on the same electronic circuit, on a graphics card or in the motherboard of a personal computer or server. GPUs and CPUs are fairly similar in construction. However, GPUs are specifically designed for performing more complex mathematical and geometric calculations. These calculations are necessary to render graphics. GPUs may contain more transistors than a CPU.
- Developers aren using GPUs as a way to accelerate workloads in areas such as artificial intelligence (AI).
- Thermal Design Power (TDP):
- TDP stands for Thermal Design Power, in watts, and refers to the power consumption under the maximum theoretical load. Power consumption is less than TDP under lower loads. The TDP is the maximum power that one should be designing the system for.
- Data Center Infrastructure Management (DCIM Software):
- DCIM software is used to measure, monitor and manage the IT equipment and supporting infrastructure of data centers. This enables data center operators to run efficient operations while improving infrastructure design planning. DCIM software can be hosted on premises or in the cloud.
- Enables organizations to track their key metrics such as database performance, energy efficiency, power consumption, and disc capacity. A thorough analysis of such metrics provides a holistic idea of the data center operations and how they can be improved for the organization.
- Electrical Power Management System (EPMS):
- Electronic system that provides fine-grained information about the flow of power in an electrical power generation system or power substation. EPMS record and provide data about power systems and power-related events.
- The EPMS monitors the electrical distribution system, typically providing data on overall and specific power consumption, power quality, and event alarms. Based on that data the system can assist in defining, and even initiating, schemes to reduce power consumption and power costs.
- BIM (Building Information Modeling):
- BIM is a set of technologies, processes and policies enabling multiple stakeholders to collaboratively design, construct and operate a Facility in virtual space. As a term, BIM has grown tremendously over the years and is now the ‘current expression of digital innovation’ across the construction industry. It is an intelligent 3D model-based process that usually requires a BIM execution plan for owners, architects, engineers, and contractors or construction professionals to more efficiently plan, design, construct, and manage buildings and infrastructure.
- BIM is a digital representation of physical and functional characteristics of a facility. A BIM is a shared knowledge resource for information about a facility forming a reliable basis for decisions during its life-cycle; defined as existing from earliest conception to demolition.
- BIM is used for creating and managing data during the design, construction, and operations process. BIM integrates multi-disciplinary data to create detailed digital representations that are managed in an open cloud platform for real-time collaboration.
- BIM process that includes not only 3D modelling, but also planning, designing, constructing, collaboration, and more. The ability to share relevant data with all of the project’s participants makes BIM an excellent collaboration tool in general.
- BEM (Building Energy Management System):
- Computer-based system that monitors and controls a building’s electrical and mechanical equipment such as lighting, power systems, heating, and ventilation. The BEMS is connected to the building’s service plant and back to a central computer to allow control of on/off times for temperatures, lighting, humidity, etc.
- Cables connect various series of hubs around the building to a central supervisory computer where building operators can control the building. The building energy management software provides control functions, monitoring, and alarms, and allows the operators to enhance building performance.
- Growth in technological advancement has made building energy management systems a vital component for managing energy demand, especially in large building sites. They can efficiently control 84 percent of your building energy consumption.
- => BIM vs. BEM: BIM is a database used for digital 3D building designs. BEM is used to map the surrounding area of a building or a design, from adjacent buildings and infrastructure in the vicinity to pipes, foundations and underground archeological monuments.
- BMS (Building Management Systems):
- Building Management or Automation Systems (BMS or BAS) are combinations of hardware and software that allow for automated control and monitoring of various building systems such as HVAC systems, lighting, access control and other security systems
- The hardware and software technologies of the BMS were created in the 60’s. Over the years, the BMS IT infrastructure grew organically and added layers of communication protocols, networks, and controls.
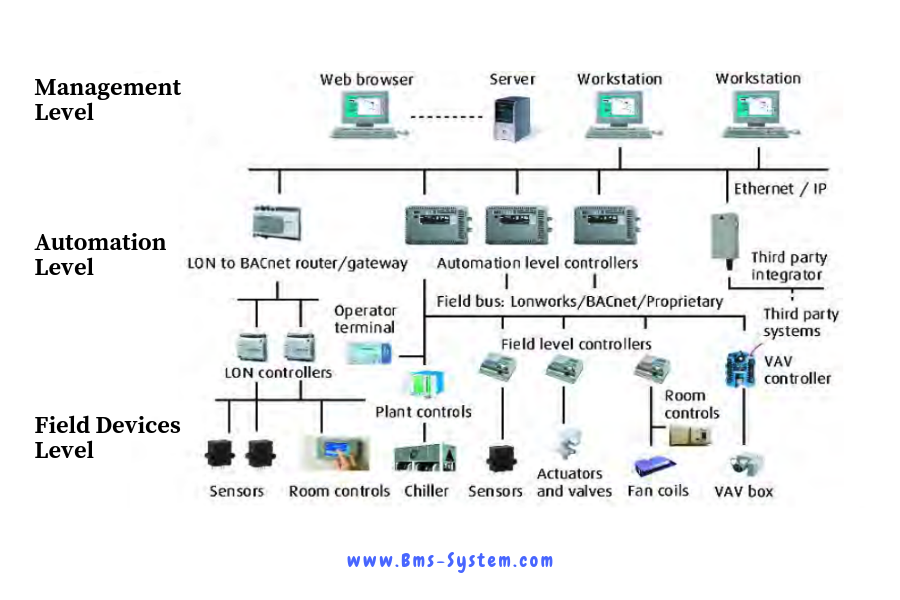
- Digital Twin:
- Digital Twin is most commonly defined as a software representation of a physical asset, system or process designed to detect, prevent, predict, and optimize through real time analytics to deliver business value.
- A digital twin is a virtual representation of an object or system that spans its lifecycle, is updated from real-time data, and uses simulation, machine learning and reasoning to help decision-making.